Confirmation Bias in Data Analysis
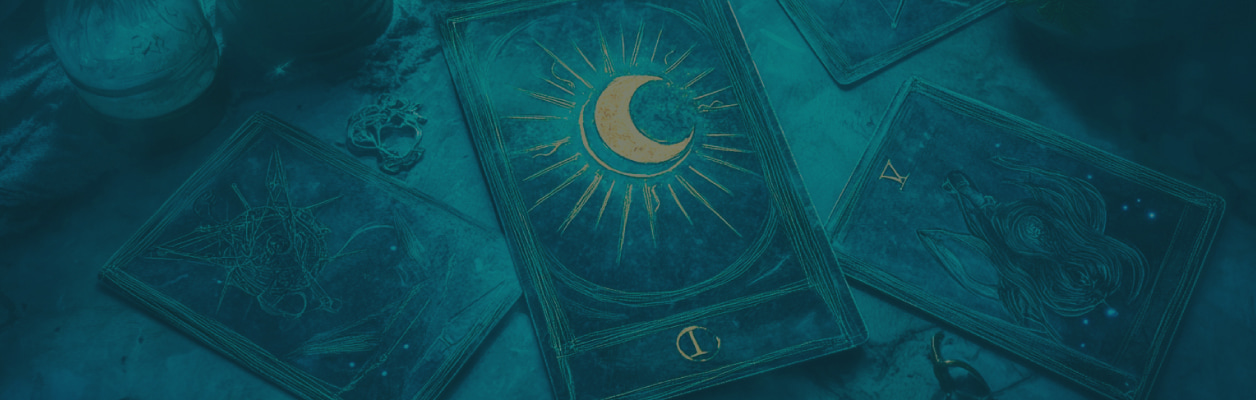
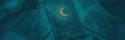
In today's data-driven world, companies rely heavily on data analytics to guide business strategies. However, the interpretation of data does not automatically yield sound decisions; this task falls to analysts who may be influenced by cognitive biases. The assumption that high-quality data analysis directly leads to effective management decisions overlooks the critical stage where analysts interpret data, where biases like confirmation bias can distort outcomes.
Understanding Cognitive Biases
Confirmation bias is part of a broader spectrum of cognitive biases, systematic errors in thinking that affect the decisions and judgements we make.
There are various classifications and several types of cognitive biases. Here are some examples:
- Fundamental attribution error (FAE): Overemphasizing personality-based explanations while underrating situational influences.
- Affinity bias: Tendency to feel attracted to, and agree with, people more like ourselves.
- Implicit stereotype: Positive or negative preconceptions we unconsciously attribute to various groups.
- Self-serving bias, due to which we may interpret ambiguous information as beneficial to us.
- Framing: Narrowing the description of a situation so that it leads to a desirable conclusion.
- Anchoring Bias: Initial data points can skew the analysis of subsequent data.
- Hindsight bias, known as the "I-knew-it-all-along" effect.
- Physical attractiveness stereotype, etc.
Among these, confirmation bias stands out because it can:
- Lead to selective data collection, where analysts focus only on data supporting their initial hypothesis.
- Cause analysts to ignore or discredit data that contradicts their beliefs, viewing them as anomalies.
- Result in misinterpretation of data, like confusing correlation with causation when it aligns with preconceived notions.
Historical Context
Notably, confirmation bias has played a critical role in significant historical events. For instance, during the 2007-2008 financial crisis, analysts and investors often overlooked or downplayed data that contradicted their optimistic forecasts for housing markets, leading to widespread misjudgment.
Psychological Underpinnings
This bias is largely driven by cognitive dissonance, where individuals prefer to maintain their belief systems for psychological comfort, and the need for cognitive consistency, where new information is molded to fit existing frameworks of understanding.
Quantitative vs. Qualitative Data
Confirmation bias can manifest differently in qualitative and quantitative data. Quantitative data might be cherry-picked to support a hypothesis, while in qualitative analysis, biases can influence how narratives are interpreted or how interviews are conducted and summarized.
Examples of Confirmation Bias
- Selective Data Collection: Analysts might only gather data that confirms their hypothesis, such as focusing on metrics showing increased customer engagement while neglecting conversion rates.
- Ignoring Contradictory Data: If one experiment out of many contradicts a theory, it might be dismissed rather than explored further.
- Echo Chambers: Within teams, biases can be reinforced, creating a collective confirmation bias where alternative viewpoints are not considered.
- Algorithmic Bias: If training data or the developers' assumptions are biased, machine learning models can perpetuate these biases.
- Framing of Questions: How a research question is asked can bias the analysis towards confirming the expected outcome.
- Data Visualization: The selection of visualization methods can amplify or minimize trends to fit a narrative.
- Literature Reviews: Researchers might give undue weight to studies that confirm their hypothesis.
- Cultural or Organizational Bias: An organization's culture can bias what data is deemed important or how it's interpreted.
- Overgeneralization: Broad conclusions might be drawn from limited data if they support pre-existing beliefs.
Strategies to Mitigate Confirmation Bias
To combat confirmation bias, consider these approaches:
- Search for Disconfirming Evidence: Actively look for data that challenges the initial hypothesis.
- Data Triangulation: Use multiple data sources and methods to validate findings. If different methods converge on the same conclusion, confidence in that conclusion increases.
- Blind Analysis: Analyze without knowing the expected outcome to reduce bias.
- Expert Assessment: Have independent experts review the analysis for bias.
- Reproducibility: Ensure that the results can be replicated by others.
- Team Diversity: Involve analysts from varied backgrounds to bring different perspectives to the table.
- Devil's Advocate: Assign someone to challenge the team's conclusions.
- Phased Decision-Making: Implement decisions in stages, allowing for iterative feedback and correction.
Practical Application
For instance, when analyzing customer feedback, a company might employ triangulation by comparing survey data with consumer interviews and social media sentiment analysis. If all sources point to a similar conclusion about product issues, this triangulation strengthens the validity of the findings. Similarly, in drug research, employing blinded analysis where data processors are unaware of expected results helps prevent biased interpretations.
By adopting these strategies, businesses and researchers can navigate the pitfalls of confirmation bias, leading to more objective and reliable decisions.
Technology and Tools
Modern data visualization tools can help analysts to mitigate biases by providing multifaceted and comprehensive representation of data. For instance: Megaladata detects missing values and outliers, thus highlighting potential sources of bias. If certain attributes are missing for a considerable number of samples, this may indicate under-representation or biased data collection. Megaladata also offers efficient mechanisms to automate bias detection, such as advanced data cleaning and distribution analysis. In Megaladata workflows, you can setup autodetecting anomalous patterns, which may be caused by cognitive biases. For example, you can create filters and calculated fields to analyze correlation and test hypotheses using statistical criteria, which allows you to avoid subjectivity.
By analyzing datasets for missing or unexpected feature values, Megaladata can highlight potential sources of bias. For instance, if certain features like temperament are missing for a significant portion of examples, this might indicate under-representation or bias in data collection.
Future Directions
As AI and machine learning continue to evolve, there's potential for these technologies to automatically detect or reduce confirmation bias. Ongoing research in cognitive psychology will likely offer new strategies for analysts to maintain objectivity in their work.
Megaladata allows you to implement algorithms of data quality assessment, to automatically determine the level of bias in different hypotheses and workflows. Using factor analysis techniques, you can discover hidden dependencies between variables. Machine learning components can develop alternative interpretations of data, which helps to combat the influence of subjective notions. By adopting these strategies and understanding the variety of cognitive bias, businesses and researchers can navigate its pitfalls, leading to more objective, reliable, and ethical decisions.
See also
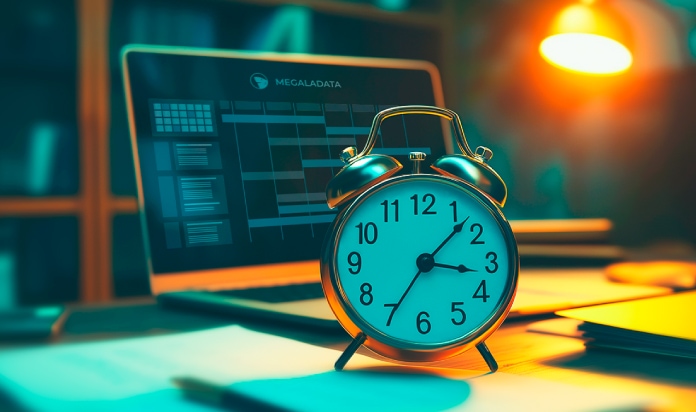
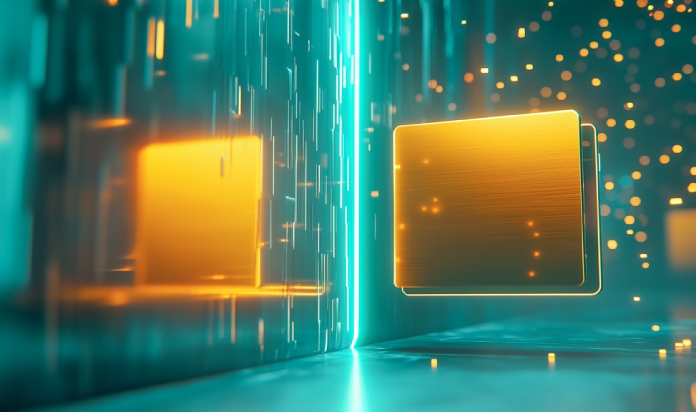
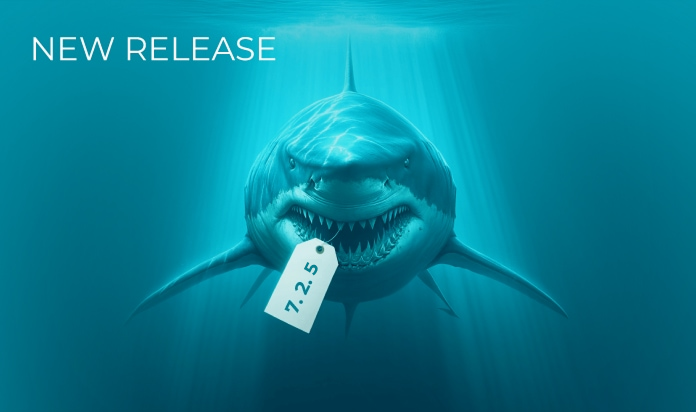