Improving Employee Skills in Data Science
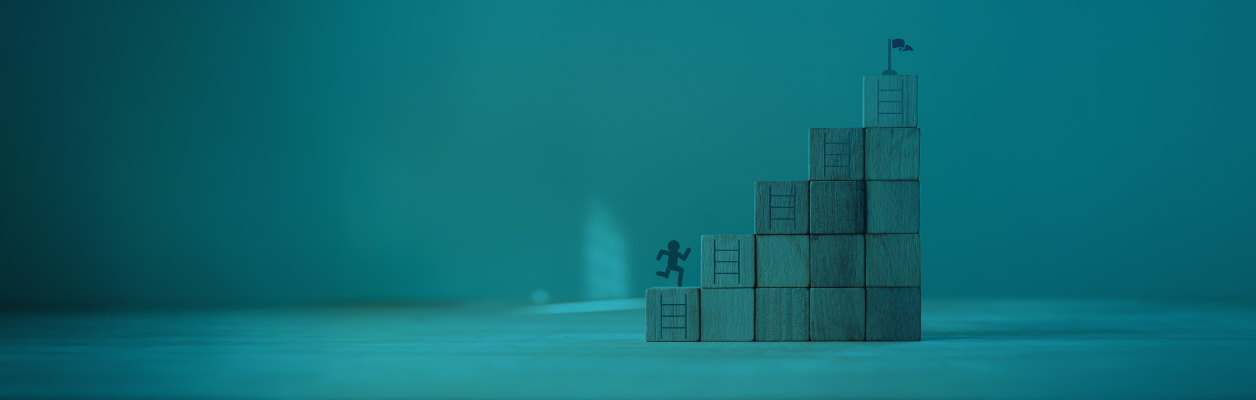
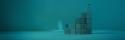
Many organizations cannot achieve their goals due to a lack of skilled personnel in Data Science. This skills shortage is expected to impact 'nine out of ten organizations by 2026 with a cost of $5.5 trillion in delays, quality issues and revenue loss', according to the International Data Corporation.
The Importance of Data Literacy
According to the Data Literacy Project data literacy 'is set to be the most in-demand skill by 2030, with 85% of executives believing these skills will be as vital in the future as the ability to use a computer is today'.
Data Literacy involves understanding data sources, reading and writing data, and communicating data insights clearly. By developing data literacy skills, employees can make informed decisions, improve efficiency and effectively communicate data insights to large audiences.
In businesses that promote data literacy across teams, it’s not just the data scientists or analysts who benefit — everyone does. Marketers can better measure their campaigns, salespeople can make more informed pitches, and leaders can make decisions based on facts, not just hunches. Companies that are good with data are more flexible, more productive, and less likely to make mistakes like misunderstanding customer trends or wasting money.
The Flywheel Effect in Data Science
We can also look to the Flywheel Effect to enhance data science learning. This self-reinforcing cycle can be initiated by a small group of early adopter data enthusiasts within a company, who possess a strong appetite for learning and a knack for applying data to solve problems. As these individuals gain proficiency, they share their knowledge through various channels, including joint data exploration sessions, and collaborative data visualization projects. These activities not only disseminate knowledge but also cultivate a sense of community and shared purpose. As more team members become confident in using data for decision-making, the cycle accelerates, fueling further skill development and knowledge sharing. By empowering more team members to harness the power of data, the flywheel effect can propel organizations towards a data-driven culture that drives innovation, reduces costs, and enhances customer experiences.
How to Achieve Effective Results
Companies should tailor their approach to their specific needs to effectively upskill employees in data science. One of the strategies is formal training, which can be delivered in-house, externally, or online. In-house training allows for customized programs led by internal experts, while external training provides access to industry best practices and specialized knowledge. Online platforms offer flexible learning options through webinars and courses.
Another approach is to foster a culture of knowledge-sharing and mentorship. Experienced employees can guide and support less experienced colleagues through mentoring programs. Additionally, organizing knowledge-sharing sessions can facilitate the exchange of insights and best practices.
To bridge the gap between theory and practice, companies can develop experiential learning opportunities. Secondment and shadowing programs allow employees to gain firsthand experience in different departments and learn from experienced colleagues. Practical projects can also provide a hands-on approach, letting people apply theoretical knowledge to real-world challenges.
To maximize the effectiveness of these strategies, companies should:
- Assess Current Skill Levels: Conduct a skill gap analysis to identify specific training needs.
- Prioritize Continuous Learning: Encourage ongoing professional development and allocate dedicated learning budgets.
- Leverage Technology: Provide access to data science platforms, tools, and collaborative workspaces.
- Foster a Data-Driven Culture: Promote data literacy and encourage data-informed decision-making.
- Measure and Evaluate: Track the impact of training programs and collect feedback to improve future initiatives.
By combining these strategies and fostering a data-driven culture, companies can empower their workforce with the necessary Data Science skills to stay competitive in the data-driven era.
The Role of Low-Code Tools
One of the most effective ways to overcome the data literacy issue is to use low-code data analysis tools. These tools can enable individuals with varying technical expertise to extract insights from data. By simplifying complex tasks and reducing the learning curve, low-code tools can empower a broader range of employees to contribute to data-driven decision-making. Low-code analytics and development is rapidly gaining popularity and attention due to its lower entry barrier and accessibility to business users and field experts. Read more about this approach in our blog post "Low-Code Philosophy as a Reason for Myth Making".
By investing in data skills training and adopting user-friendly tools, organizations can unlock the full potential of their data and drive innovation. Megaladata provides a non-commercial Community Edition, which anyone can explore free of charge, to gain understanding of the low-code approach and experience the platform's unprecedented performance and ease of use. Feel free to compare it to other available analysis platforms and techniques, and see if low code is the right choice for your company's upskill journey.
See also
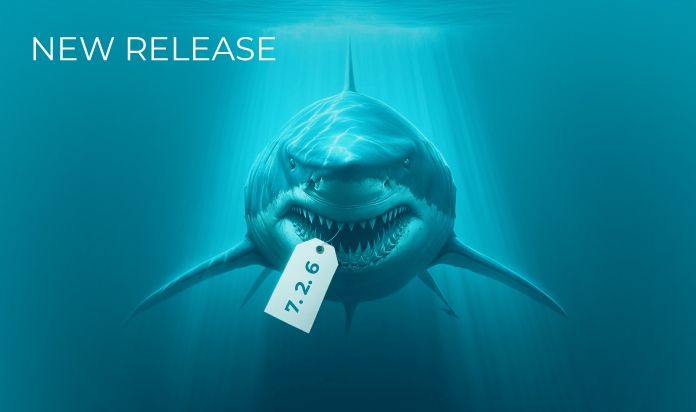
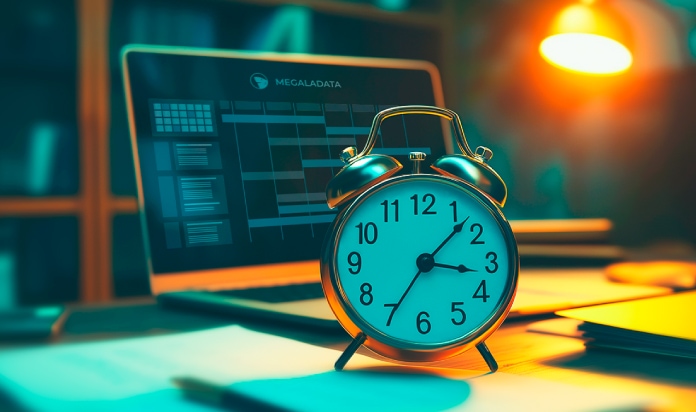
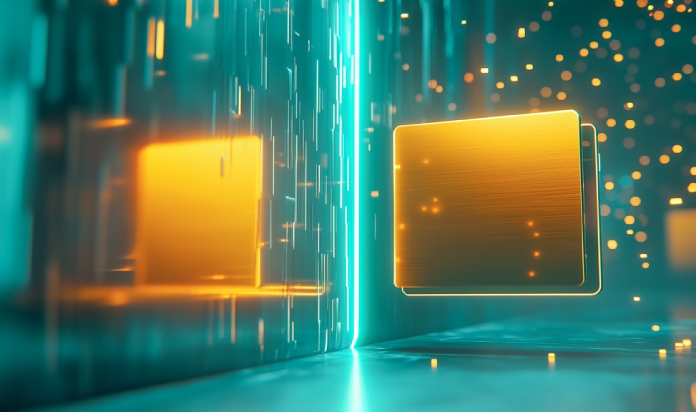