Statistics: The Foundation of Data Science
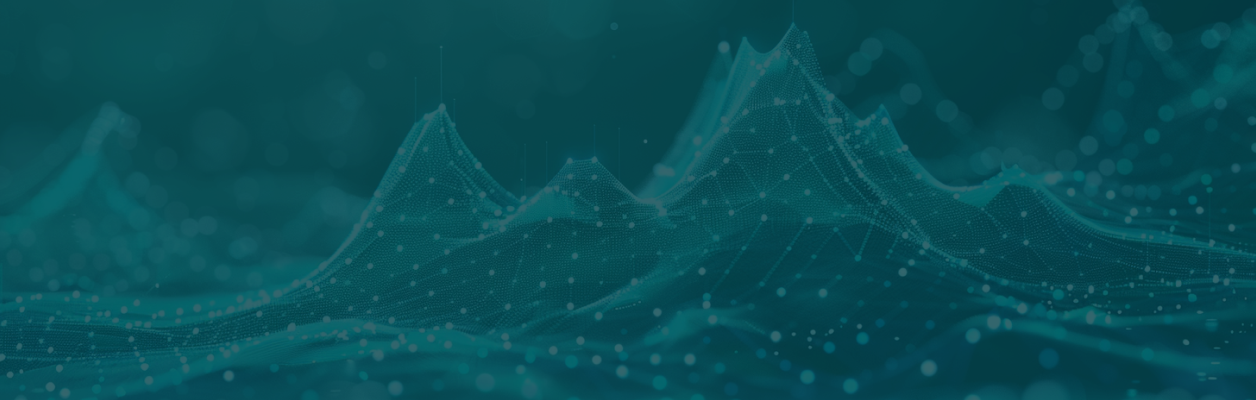
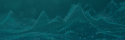
How much statistics do you need for data science?
Statistics is a familiar concept, woven into the fabric of our daily lives. From budgeting and travel planning to price comparisons and scheduling, we instinctively apply statistical thinking. Formally, statistics is the art of extracting insights from data. By employing various statistical methods, we can manage vast datasets, compare groups, replicate research, and effectively communicate findings to diverse audiences. However, it's crucial to remember that a statistical result is merely a number, requiring careful interpretation. While a valuable tool, statistics alone cannot provide definitive answers.
Here are some concepts in statistics that can help you interpret your data
Descriptive Statistics focuses on collecting, summarizing, and presenting a dataset.
Examples: The average age of citizens who voted for the winning candidate in the last presidential election, the average length of all books about statistics, and the variation in the weight of 100 boxes of cereal selected from a factory’s production line.
Interpretation: You will likely be familiar with this branch of statistics because many examples arise in everyday life. Descriptive statistics form the basis for analysis and discussion in such diverse fields as securities trading, the social sciences, government, the health sciences, and professional sports.
Inferential Statistics is the branch of statistics that analyzes sample data to conclude a population.
Example: calculating the average height of all adults in a city
To estimate the average height of all adults in your city, you'd use inferential statistics. You'd measure a sample of people, calculate their average height, and then use that to predict the average height of the entire population.
Interpretation: When you use inferential statistics, you start with a hypothesis and look to see whether the data are consistent with that hypothesis. Statistical methods based on inference often lead to misapplication or misinterpretation.
Probability is a numerical measure of the likelihood that an event will occur. It's expressed as a number between 0 and 1, where:
- 0 means the event is impossible
- 1 means the event is certain
Example: winning the lottery
Lotteries offer extremely low probabilities of winning. Let's consider a simple lottery where you pick 3 numbers from 1 to 10.
- Total number of possible outcomes: 10 * 10 * 10 = 1000 (assuming you can pick the same number multiple times)
- Number of favorable outcomes (matching all 3 numbers): 1
Interpretation: Therefore, the probability of winning this lottery is 1/1000 or 0.001. This means there's a 0.1% chance of winning, that highlights the very low likelihood of such an event.
Regression analysis is a statistical method used to examine the relationship between variables. It helps us understand how changes in independent variables affect a dependent variable. Essentially, it's about finding patterns in data and building a mathematical model to predict outcomes.
Example: predicting the price of a house
We can use regression analysis to determine how factors like size, location, number of bedrooms, and bathrooms influence the price. By analyzing data on sold houses, we can create a model that estimates the cost of a new one based on its characteristics.
Interpretation: Once we have a regression model, we can interpret the results to understand the relationship between variables. For example, if the coefficient for house size is positive, it means that as the size increases, the price tends to increase as well. We can also calculate how well the model fits the data to assess its accuracy in predicting house prices.
Conclusion
As data science projects grow in complexity, so does the need for statistical proficiency. As your curiosity and the complexity of your work grow, your statistical skills will become increasingly important. Megaladata can be a valuable asset in this journey, providing a platform for learning and applying statistical concepts. It also offers visualizations like histograms and scatter plots. For deeper analysis, components for regression, correlation, factor analysis, and autocorrelation are available. Download the free Megaladata Community Edition and explore its capabilities, all while enjoying the unrivaled computational speed!
See also
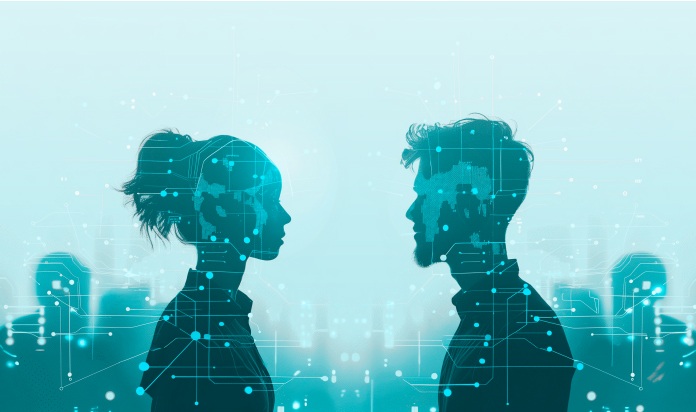
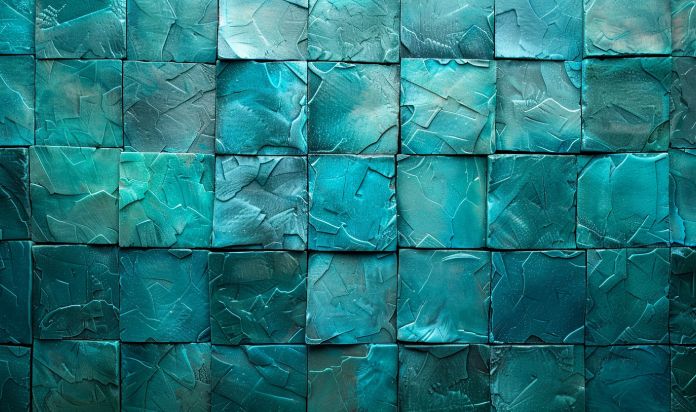
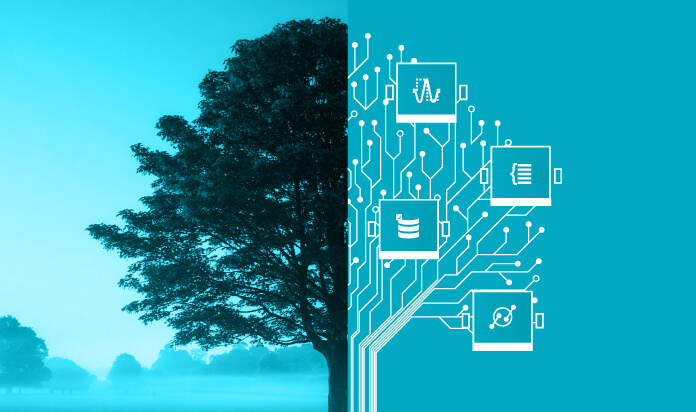