Customer segmentation by loyalty or RFM analysis
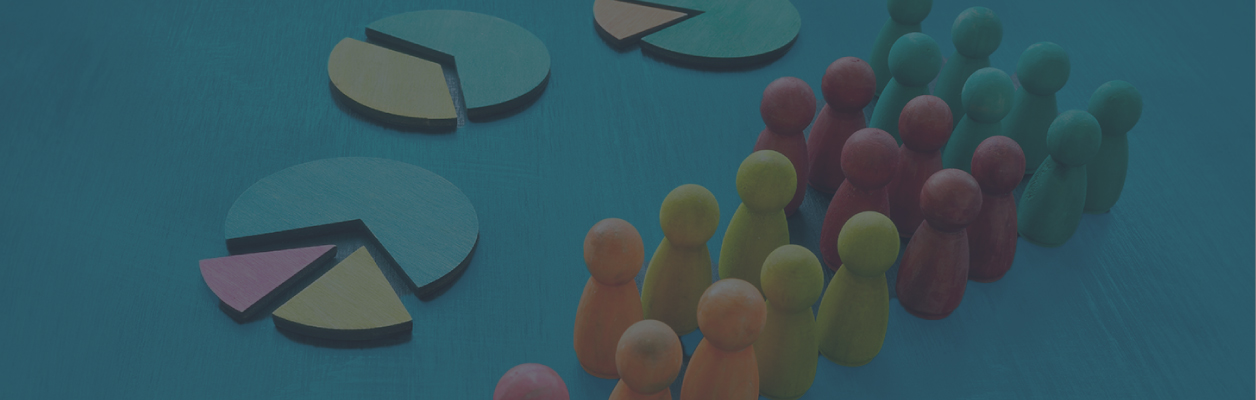
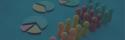
There are two types of data that you can learn about customers: who they are (demographic data) and what they do (behavioral data). As a rule, marketers try to predict the behavior of customers. After all, if you are planning to sell a product or service to your customers, then knowing that one of them recently purchased several items with a total value of 10,000 rubles is more valuable than knowing their age, income, marital status or other similar information.
Direct marketing specialists usually use RFM analysis to predict customer behavior. The main question is how to formalize the amount of benefit brought by each client. Three indicators are being studied for this purpose.
- Recency — the time limitation of the sale (how much time has passed since the client made their last purchase)
- Frequency — the periodicity of purchases (the total number of purchases in the period)
- Monetary — the total amount of sales (sometimes the average check is used)
Notably, the Monetary indicator does not have to be linked to money. This can be any filter applied to the main indicators of this method: the Recency and Frequency. It is not always possible to reduce monetization to purchases. For example, for an information portal, you can take the page view time or the page depth of the site.
As a rule, the percentage of buyers who respond to general advertising offers is small. RFM analysis is a simple and, at the same time, efficient method. You can use it to predict the reaction of a buyer, improve interaction with customers and increase profits. Depending on whether the clients belong to one of the selected groups, you can use different methods to influence them. The most importance of indicator is Recency, followed by Frequency and, finally, the Amount of Sales.
Applying RFM to a private client database
Let us consider a small textbook sales company that used RFM analysis to make a maximum profit on each customer. Every summer, it sent letters to all its customers, suggesting they should purchase the company's products. The cost of mailing was 15 rubles per letter, while the profit from each sale was 550 rubles. Thus, to justify the cost of mailing, the company needed to receive over 3% of responses. But for several years, the response rate failed to exceed 1.6%, which brought no profit. With the help of RFM, the entire existing customer database was divided into 125 cells (5x5x5). The response rate for each of the cells was determined. Later on, the company began to carry out its mailing within the cells which have a response rate of over 3%, enabling it to eventually make a profit.
It is worth noting that the business will not benefit from the continuous use of RFM analysis. You might use it to constantly answer the question: which client to write or call. But then, some clients will never hear from you at all. Others, who react the most actively, will experience fatigue from the constant attention because you will contact them too often. You need to develop a contact strategy and think about what kind of offer you can make to less receptive customers. These ones need to know that you remember about them.
In practice, there is a simplified RFM analysis, which is called RF segmentation. In this case, the Sales Amount indicator is not used because its value often depends on the Frequency. It is easy to identify which customers you need to actively work with through this analysis and develop special offers for them.
Applying RFM to a corporate customer database
Consider the application of RFM analysis to a database of corporate clients. This kind of database is, in most cases, much smaller than a database made up of private clients. So you can resort to reducing the number of groups. For example, for a database of 10,000 records, 125 RFM analysis cells will have only 80 records in each cell. This is not enough to get statistical results. Let us reduce the number of RFM cells to 20 with a decreased number of groups sorted by Frequency and Amount of Sales. For example, 5 by the Date of Purchase, 2 by Frequency and 2 by the Amount of Sales.
The theory of RFM analysis assumes a very simple mechanism for dividing the Recency, Frequency, and Amount of Sales by segment. You sort all contacts and divide them into 5 equal groups for each indicator. This method is productive and helps to break all customers in relation to each other into the following groups.
- Just bought
- Bought recently
- Bought a long time ago
- Bought a very long time ago
But in practice, there is a problem because these indicators change over time.
Example 1
Business is developing well but suddenly not a single purchase is made for a month. This is bad. Yet if you split the database into equal parts, nothing will change. The recent buyers will still fall into the Just Bought segment.
Example 2
The new business does not yet have sales data for several years. In this case, the boundaries will be very different every day and there is no point in using the result of such an RFM analysis at all.
Seasonal patterns, promotions and holidays also strongly affect the quality of the analyzed data. Therefore, you should set a proper time. To determine which time intervals you should set, it is worth answering a number of questions.
- What is the length of the customer lifecycle?
- What is the natural purchase period?
- What are the seasonal patterns?
- How long does it take for most customers to make a repeat purchase?
The efficiency of RFM analysis does not mean that it can completely replace the sales manager. Personal communication with the customer enables the employee to understand them well and know a lot about them. The right thing to do is to focus the manager's attention on the key customers and work with the rest of the customers via email, messages and social networks. In this case, RFM analysis becomes an excellent tool for segmenting customers.
See also
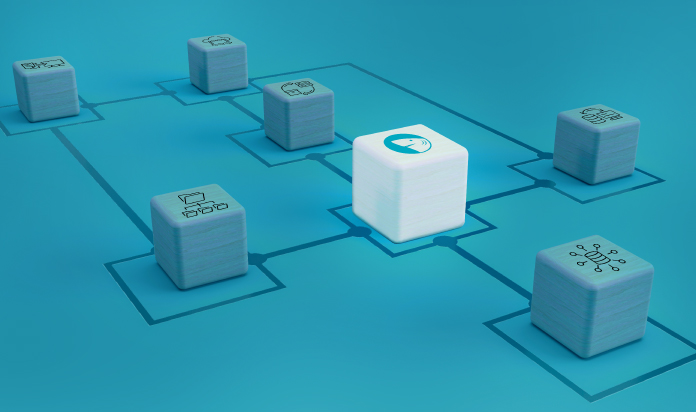
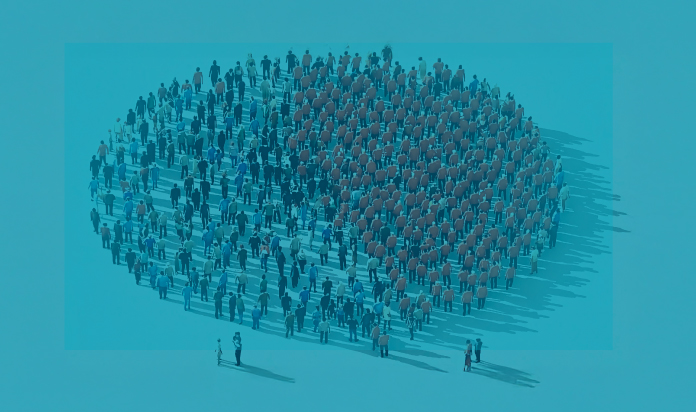
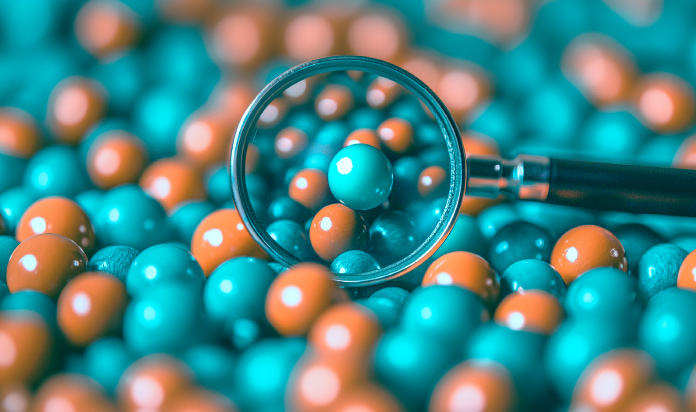