Shewhart Charts as a Tool to Control Business Processes
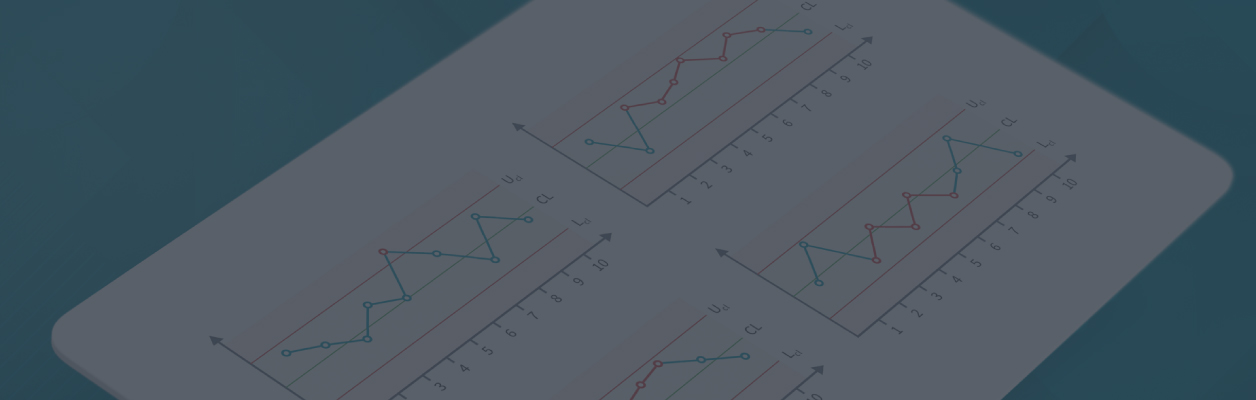
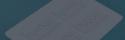
In the 2010-11 fiscal year, the American technology corporation Nvidia faced losses caused by the mass production of defective GPUs. Faulty manufacturing of a single detail led to significant troubles for the whole business: the low-quality packages made the microchips overheat and break quickly. Repair and replacement cost the company $315 million - 12 times the regular maintenance costs.
Is it possible to avoid such situations in modern manufacturing, which consists of thousands of processes? Naturally, solving such problems post-factum leads to an abrupt increase in costs. It is only predictive analytics that can help.
When all the processes are stable, their future behavior is predictable. It makes business management way less burdensome. For example, if we know the approximate future workload, pre-calculating the number of staff required takes much less effort.
The same applies to the manufacturing machinery. Knowing the planned production load, we can evenly distribute it over the equipment, avoiding downtime and overload, which both result in financial loss.
How to predict the behavior of a process?
The answer was given in 1924 by the American scientist Walter A. Shewhart. He is sometimes called the father of modern quality control. Studying statistics, Shewhart was determined to use it for the good of the manufacturing industry.
As a result, he developed a specialized graphic tool that allows for tracking deviations from normal behavior and error tendencies before actual manufacturing defects appear. It was a breakthrough in the area of quality control.
Initially, Shewhart's control charts served to prevent production defects. But the tool proved to be universal, fitting any processes of a regular nature. It is now widely adopted in all business fields. For example, in services, customer complaints can be considered defects.
What Are the Control Charts?
Shewhart control charts are graphs of process parameter changes used to perform statistical control of the process's stability.
The main goal of the chart is to determine whether a sequence of data can be used to predict the future behavior of the process. If the data doesn't fit this purpose, the chart indicates that the process is unstable. If the data is applicable, it serves as the basis for the forecast.
In this approach, the main criterion of a process is its stability, which relates directly to the causes of variation.
There are two types of such causes:
- special (assignable cause): External influence on the system, unstable process.
- common (chance cause): It is an inherent part of the system, so the process is considered stable.
Control charts serve as a means of eliminating special-cause variation. The process then becomes stable and predictable. Its quality rises, and the costs decrease.
The vertical axis of the chart represents multiple values of a process parameter. Along the horizontal axis, the analyst plots the numbers of the samples for which the statistic is measured.
It is important to note that the method applies to regular processes (recurring after a certain time), as you need many observations of the statistic to build a chart.
The figure below is an example of a control chart.
Сenter line (CL) represents the base value of the statistic — usually the mean value. Depending on the managerial goal, it can also be a planned (desired) value.
Upper and lower control limits (UCL and LCL) are the lines drawn above and below the center line. They indicate the thresholds for the parameter. While the value of the statistic stays within the range, the process is considered stable.
It is critical to set the control limits correctly. If they are placed too close to the center line, the chart will signal problems when there are none. However, if the range is too broad, some special causes of variation may remain unidentified.
Shewhart suggested drawing the limits at 3-sigma from the center line, with sigma being the true value of the standard deviation in the process. For a normally distributed statistic, when the process is in control, 99.7% of all the points will fall between the control limits.
As the control charts detect and indicate the points when the process becomes unstable, it is a common practice to add special 'warning' limits at a 2-sigma distance from the center line. Crossing these lines signals that the process may come out of control limits soon.
A significant advantage of Shewhart charts is their non-sensitivity to minor changes in the process. That is, they 'react' only to abnormalities that indeed cause the unstable process behavior.
Types of Control Charts
Shewhart charts can be classified based on the type of data they represent:
- Quantitative data: Measurement of the outcomes within the process.
- Binary data: Characterized by discrete values (yes/no).
Charts of the first type are more commonly used, despite the more challenging data collection.
Advantages of qualitative data control charts:
- Most processes have characteristics that are measurable.
- A measurement provides more information than a simple yes/no statement.
- The data can be analyzed independently of the set requirements.
For each chart, the control values for the statistic of the process may be:
- not specified. In this case, the data analysis is based on the values that appear in the process itself. The analyst identifies variations that can stem only from special causes.
- specified. Such values are usually taken from the requirements or obtained by analyzing the data over a long period of time. In this case, the analyst compares the observed values to the given ones.
The difference between these two types of charts lies in the presence of additional criteria for setting the center line and control limits of the second-type charts.
Plotting a Control Chart
Upon initial plotting of a chart, it is not uncommon to discover that the process is out of statistical control and should be brought to a state of stability.
The construction of a chart has two stages:
- Identifying the statistics for the control chart. A large amount of data is collected and processed to get empirical values of the center line and the limits of the process. These values are considered test data, as the process is not certainly in statistical control at the moment of collection. The analyst must repeatedly investigate special-cause variations until the chart stops signaling their presence — which confirms that the process has become stable.
- Maintaining the state of statistical control. The values resulting from the first stage become the chart's control parameters in the second stage. The main goal now is to keep the process in control and quickly detect special causes that interfere with it.
Main rules for detecting instability:
- Any point appearing outside the control limits.
- A run of 7 or more points all above or below the center line.
- A run of 7 points going successively up or down.
- An area where changes do not seem to be random.
For initial chart analysis, it is recommended to follow the first rule, introduced by Shewhart himself. The rest of the rules can be added if required, depending on the nature of the process.
In addition, long-term analysis of the process often leads to the discovery of unique out-of-control indicators that can also be taken into account. However, it is necessary to remember that every additional criterion makes a 'false alarm' more probable.
Efficient Business Process Management
As business processes are prone to variation, they require constant control and rapid decision-making in case of a loss of stability.
For single operations, it is possible to perform the control manually. However, when we deal with regular processes, the amount of information to analyze is so large that the need for automation becomes obvious.
For example, a bank receives thousands of loan applications daily. Although the lending decision process is standard, variation is still present. Possessing the data from previous periods, the manager can calculate the control limits and quickly identify the points outside the range.
The figure below is a plot illustrating a real-life process. The points are chaotic; many fall out of the control range.
In the next figure, the process has become stable. All the points are within the range; some structure has appeared. It is possible to predict the future behavior of the process.
The last figure demonstrates a stage of process control and prevention of its instability. Point 7 falls out of range, signaling the need for control action.
Advantages of Using Control Charts
Here is the bottom line: Shewhart control charts are a comprehensive tool for automated process management.
They are worth using due to the following capabilities:
- Quick signaling when the process starts coming out of control. The charts allow the managers to react promptly to resolve the problem.
- Decreasing variation within the process. After the process has been brought to stability, the total number of variations for its parameters becomes lower.
- Uncovering the general tendency of the system. It gives you the opportunity to control the process and predict its behavior.
See also
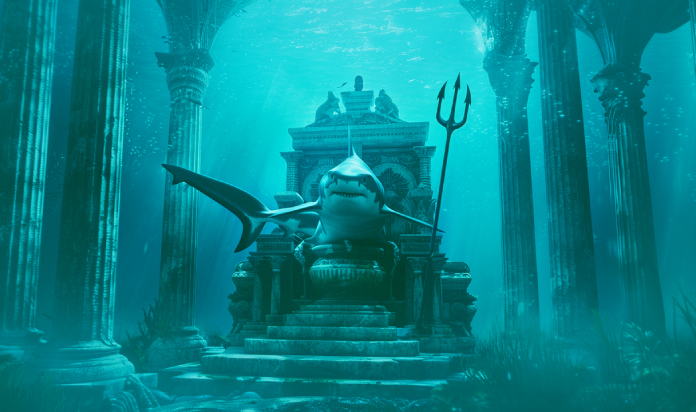
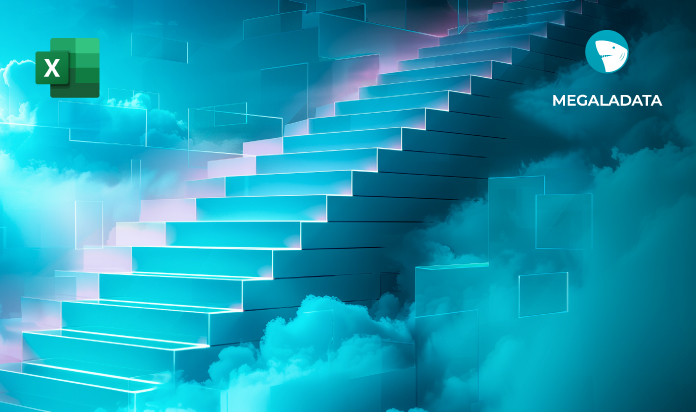
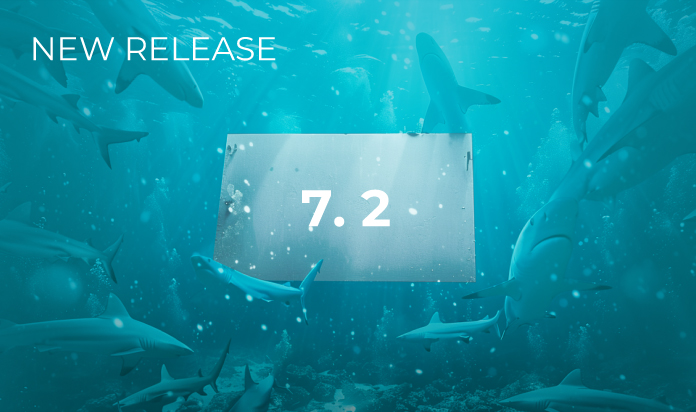